Using artificial intelligence to discover new treatments for superbugs
Antimicrobial resistance is an emerging threat to healthcare systems worldwide. As a consequence of the spread of drug-resistant bacteria, also called “superbugs,” medical treatments could become ineffective for an increasing number of people in the next years. To fix this huge problem, chemists are asked to find new effective antibiotics.
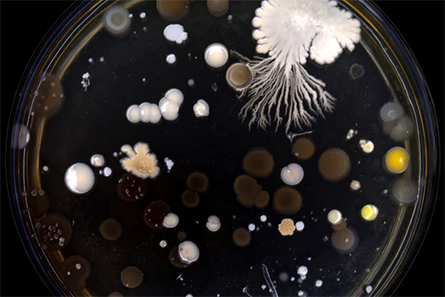
Drug discovery is an expensive and time-consuming process during which pharmaceutical chemists look for new candidate molecules to interact with a particular target protein or pathway causing the disease. Chemists screen large libraries of thousands to millions of molecules, looking for compounds with specific biological effects and low toxicity. However, these screenings are not very efficient: if chemical libraries don’t include molecules with enough structural diversity, chemists will fail to discover antibiotics with molecular structures different from the ones already tested in laboratories or clinical trials.
Now machine learning is flanking chemoinformatics through innovative deep neural network approaches to find new drugs. An example of how this approach works can be seen in a recent study by James Collins and coworkers at MIT. First, researchers trained a neural network model to predict growth inhibition of Escherichia coli using a set of 2335 diverse molecules; then, they applied the optimized neural network model to screen large chemical libraries with more than 107 million molecules.
They ended up with a list of candidate molecules structurally different from known antibiotics, and ranked them based on their predicted biological activity. Among those candidates, they found that halicin, a compound under investigation as a treatment for diabetes, displayed high efficacy against E. coli and a large spectrum of pathogens such as Acinetobacter baumanii, at the top list of resistant bacteria which urgently requires new antibiotics.
Research groups are currently developing similar deep learning approaches to find new compounds that could fight the COVID-19 virus. This suggests how recent improvements in machine learning can assist chemists’ work to speed up and lower the costs of the drug discovery process.
This story originally appeared on Massive Science, an editorial partner site that publishes science stories by scientists. Subscribe to their newsletter to get even more science sent straight to you.

Enjoy reading ASBMB Today?
Become a member to receive the print edition four times a year and the digital edition monthly.
Learn moreGet the latest from ASBMB Today
Enter your email address, and we’ll send you a weekly email with recent articles, interviews and more.
Latest in Science
Science highlights or most popular articles
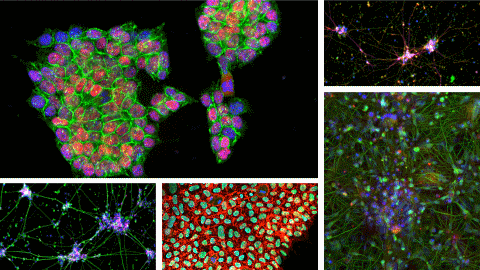
A new kind of stem cell is revolutionizing regenerative medicine
Induced pluripotent stem cells are paving the way for personalized treatments to diabetes, vision loss and more. However, scientists still face hurdles such as strict regulations, scalability, cell longevity and immune rejection.
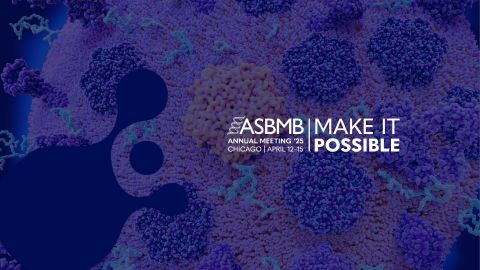
Engineering the future with synthetic biology
Learn about the ASBMB 2025 symposium on synthetic biology, featuring applications to better human and environmental health.
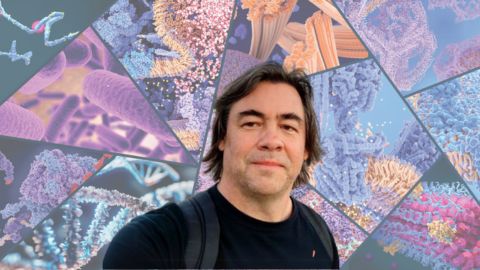
Scientists find bacterial ‘Achilles’ heel’ to combat antibiotic resistance
Alejandro Vila, an ASBMB Breakthroughs speaker, discussed his work on metallo-β-lactamase enzymes and their dependence on zinc.
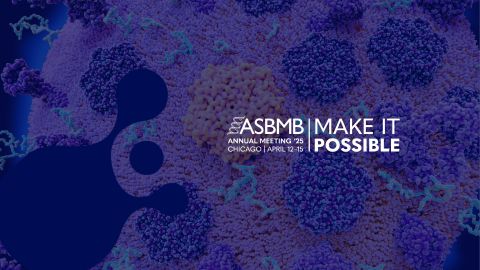
Host vs. pathogen and the molecular arms race
Learn about the ASBMB 2025 symposium on host–pathogen interactions, to be held Sunday, April 13 at 1:50 p.m.
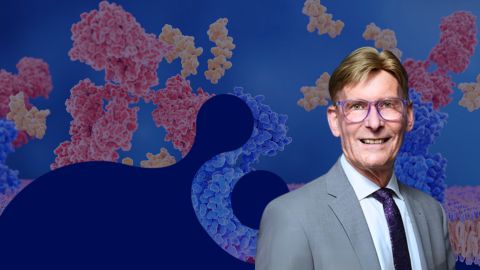
Richard Silverman to speak at ASBMB 2025
Richard Silverman and Melissa Moore are the featured speakers at the ASBMB annual meeting to be held April 12-15 in Chicago.
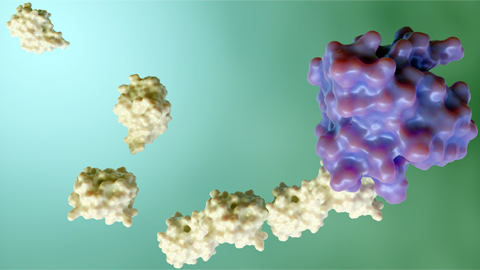
From the Journals: JBC
How cells recover from stress. Cancer cells need cysteine to proliferate. Method to make small membrane proteins. Read about papers on these topics recently published in the Journal of Biological Chemistry.