Why AlphaFold 3 needs to be open source
Imagine a world where in a matter of minutes, scientists could identify drugs to treat incurable diseases, design chemicals that could break down plastics to clean up pollution, and develop new materials that can suck excess carbon dioxide out of the air to help address climate change. This is the promise of new biology- and chemistry-based models that use artificial intelligence, or AI, to perform traditionally time-consuming tasks such as determining the structures of proteins.
Google DeepMind, a private research subsidiary of Google, released the highly anticipated AlphaFold 3 model last month as a paper in Nature. This model claims to be an improvement over its earlier version, AlphaFold 2, because it can predict not just protein structures, but also how they interact with RNA, DNA, and — most importantly — drugs. DeepMind said that it hopes AlphaFold 3 will “transform our understanding of the biological world and drug discovery.”
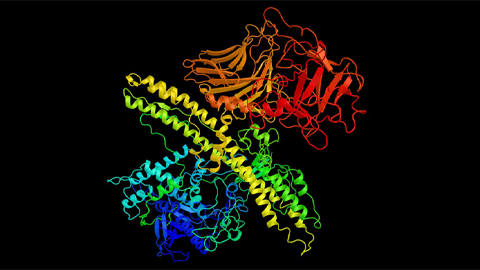
However, it’s unlikely to change how computer scientists such as myself understand biology anytime soon, because Nature, the highly competitive journal that states its mission is to “serve scientists,” allowed DeepMind to keep the software’s code unavailable, despite its own editorial policy requiring authors “to make materials, data, code, and associated protocols promptly available to readers without undue qualifications.”
In an interview with Nature reporter Ewen Callaway, DeepMind cited its own commercial interests as a reason to restrict access, in particular through its spinoff company Isomorphic Labs. “We have to strike a balance between making sure that this is accessible and has the impact in the scientific community as well as not compromising Isomorphic’s ability to pursue commercial drug discovery,” said Pushmeet Kohli, DeepMind’s head of AI science and vice president of research.
Since DeepMind did produce the software, it’s understandable that the company should be the one to determine how AlphaFold 3 gets released. DeepMind will just have to pay the consequences that its software may not be as popular among researchers.
Google CEO Sundar Pichai wrote that more than 1.8 million people have used previous versions of AlphaFold, most notably AlphaFold 2, the earth-shatteringly powerful technology released by DeepMind in 2021. A large part of its popularity came because it was verified by hundreds of academic groups, for example during the CASP14 competition in 2020, a global challenge held every two years where teams make predictions on the structures of proteins that have never been seen before.
AlphaFold 3 has no third party verification of the results it describes in the paper, leaving researchers no recourse but to believe that the model’s results are correct, presumably because they came from the creators of the highly successful AlphaFold 2.
“The amount of disclosure in the AlphaFold3 publication is appropriate for an announcement on a company website,” stated 10 scientists in a letter submitted to the editors of Nature, “but it fails to meet the scientific community’s standards of being usable, scalable, and transparent.” As of May 28, the letter has accumulated more than 1,000 signatures.
In response to the letter, Kohli quickly came out on social media stating that the model will be downloadable for academic use in the next six months. I applaud Kohli and DeepMind on this statement; however, concerns remain. A post on X is not a binding agreement between DeepMind and Nature; it contains vague release details with a deadline far in the future.
In an editorial response published on May 22, Nature claimed that by allowing peer-reviewed publications from the private sector, it “promotes the sharing of knowledge, verification of the research and the reproducibility researchers strive for” and that its policy states that the editors reserve the right to decide if all code needs to be released. However, it’s unclear to me how one can verify research without having the tools available to do so.
Popular journals such as Nature need to employ equal standards for all groups, not make exceptions for large for-profit industries. Instead, AlphaFold 3 should have been posted as a paper on bioRxiv — a widely accepted database of preprints, or non-peer reviewed articles — until all materials needed to reproduce the results were released. It could even have been just a blog post, similar to how the text-to-video model, Sora, by OpenAI, was released.
Due to widespread criticism in many academic circles, Nature Editor-in-Chief Magdalena Skipper appeared to suggest to Retraction Watch and to Science that biosecurity and ethical concerns were the reason to publish AlphaFold 3 without open-access code. This concern is understandable given that in March, leaders in the biotechnology community released a letter expressing the need to self-regulate AI.
However, DeepMind never explicitly stated that biosecurity was a reason for limiting access. I was only able to find a semi-relevant statement in the press release, which says that DeepMind worked with 50 domain experts “to understand the capabilities of successive AlphaFold models and any potential risks.”
Even if DeepMind were concerned with biosecurity, the restricted release doesn’t follow the precedent set by DeepMind itself for publishing models that could be used for unethical purposes. For example, in September, DeepMind released a model to help understand rare genetic diseases, AlphaMissense, in the journal Science, along with the code to reproduce the model.
The paper notes that the source code can be downloaded, but parts of the model were not shared to “prevent use in potentially unsafe applications.” According to MIT Technology Review, the decision was assessed by DeepMind’s responsible AI team and an anonymous “outside biosafety expert,” in order to reduce misuse of the model by bad actors. This is like giving someone the recipe to bake a cake, instead of handing them one fresh out of the oven.
Under this type of release, researchers who want to replicate the results must start over, implementing the model from scratch, which is a long and expensive process but doable with enough effort. That way, everyone wins: The model’s abilities can be assessed fairly — including identifying any unknown security concerns — but it can’t be quickly reproduced by bad actors.
If DeepMind were truly concerned about the biosecurity implications of AlphaFold 3, it should have stated that concern directly, and Nature should have demanded a code release similar to that of AlphaMissense.
Perhaps by upholding open-access standards, we will be able to achieve a perfect future, one in which all diseases can be cured, plastic pollution is cleaned up, and climate change is mitigated. However, we won’t have a chance to get there if the rules for academic publication are not applied equally.
This article was originally published on Undark. Read the original article.

Enjoy reading ASBMB Today?
Become a member to receive the print edition four times a year and the digital edition monthly.
Learn moreGet the latest from ASBMB Today
Enter your email address, and we’ll send you a weekly email with recent articles, interviews and more.
Latest in Opinions
Opinions highlights or most popular articles
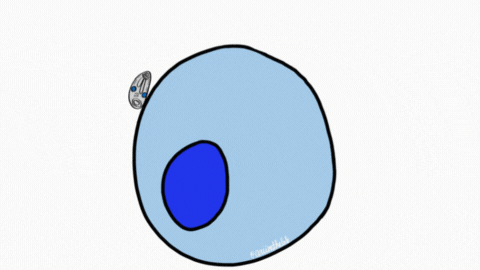
Sketching, scribbling and scicomm
Graduate student Ari Paiz describes how her love of science and art blend to make her an effective science communicator.
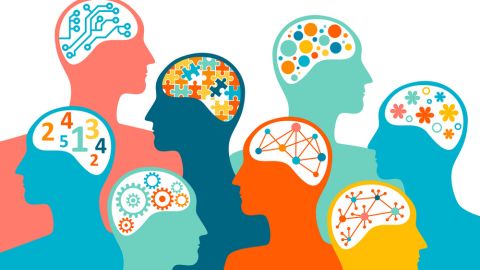
Embrace your neurodivergence and flourish in college
This guide offers practical advice on setting yourself up for success — learn how to leverage campus resources, work with professors and embrace your strengths.
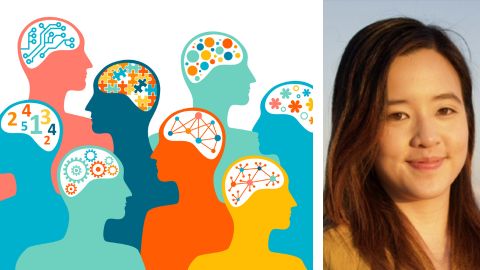
Survival tools for a neurodivergent brain in academia
Working in academia is hard, and being neurodivergent makes it harder. Here are a few tools that may help, from a Ph.D. student with ADHD.
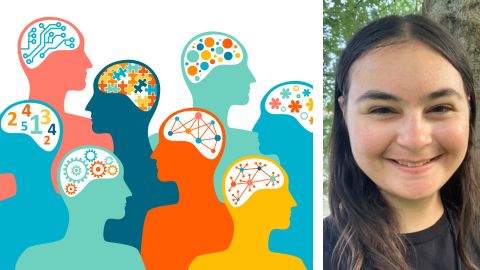
Hidden strengths of an autistic scientist
Navigating the world of scientific research as an autistic scientist comes with unique challenges —microaggressions, communication hurdles and the constant pressure to conform to social norms, postbaccalaureate student Taylor Stolberg writes.
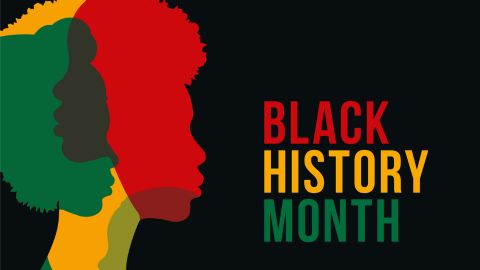
Black excellence in biotech: Shaping the future of an industry
This Black History Month, we highlight the impact of DEI initiatives, trailblazing scientists and industry leaders working to create a more inclusive and scientific community. Discover how you can be part of the movement.

Attend ASBMB’s career and education fair
Attending the ASBMB career and education fair is a great way to explore new opportunities, make valuable connections and gain insights into potential career paths.