
Machine learning lends a hand to catalyze greener chemistry
If you walk into a molecular biology laboratory and open a freezer, odds are you will find it filled with tiny tubes crowned with colorful screw-on caps and marked with names like DNA polymerase, DNA ligase, protease, reverse transcriptase, luciferase and restriction endonuclease. As their shared “-ase” suffix discloses, these faithful friends of molecular biologists everywhere are enzymes, proteins that catalyze biologic reactions. They help synthesize DNA, create plasmids, chop up proteins and measure the activity levels of gene-activating promoters as researchers strive to unlock the secrets of life. Many of those secrets involve the activity of enzymes that catalyze essential, life-sustaining chemical reactions in living cells.
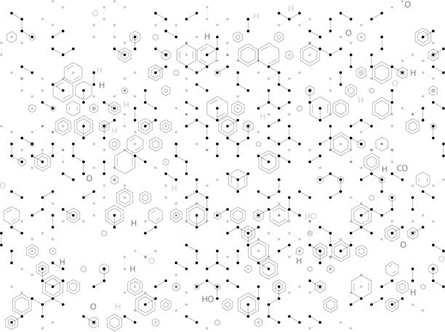
Beyond crowded cellular cytoplasm and cluttered laboratory freezers, you also can find enzymes hard at work in industry. They are key to the production of our favorite commercial food products, crisp white paper products, stain-smiting detergents and pharmaceuticals: If you’ve gotten an mRNA vaccine to protect you from COVID-19, you can thank RNA polymerase for turning a pool of loose ribonucleotides into a powerful preventative. Inspired by these successes as well as environmental and practical concerns, many researchers now are looking to harness the power of machine learning to expand the role of enzymes in industry.
Chemically speaking, enzymes are catalysts: substances that increase the speed of a reaction without themselves being consumed. Catalysts offer many advantages in manufacturing processes, including faster reaction speeds, minimization of waste products, fewer reaction steps and recyclability. As the climate warms and human-produced waste products permeate the planet, interest in promoting green chemistry through the use of catalysts has burgeoned.
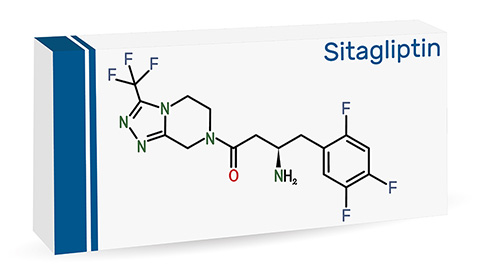
Among catalysts, enzymes are particularly attractive since they are typically easy to obtain (proteins can be synthesized by and purified from fast-growing microbes like E. coli); operate under mild, nontoxic conditions, usually in aqueous environments at body temperature; are highly efficient; and offer stereo- and regioselectivity. Advances in the manufacture of sitagliptin, a widely used Type 2 diabetes medication, illustrates the advantages of enzyme catalysis in chemical manufacturing: A transaminase obtained through directed evolution replaced an expensive high-pressure hydrogenation step, inorganic rhodium and iron catalysts, and a chiral purification step to remove unwanted stereoisomers, offering improved yield and reduced waste.
However, there often isn’t an obvious choice for catalyzing a given reaction, especially if it normally doesn’t occur within a living organism. In the sitagliptin example, initial testing of known transaminases yielded none with the desired activity. It took additional tests on a truncated version of the substrate to uncover a candidate with even a tiny amount of activity and then an extensive directed evolution process to compound this activity until the final transaminase was obtained. Even if one is prepared to embark on such an endeavor, deciding where to start requires deep knowledge of all existing enzymes, their substrates and their stereo- and regioselective properties.
Enter machine learning. Quickly becoming the natural choice for tackling any data set that is too complex and vast for a human brain to analyze fully, machine learning — a type of artificial intelligence — involves training a computational framework by exposing it to data related to a task of interest so it can learn patterns needed to process new material properly. For example, a model whose goal is to recognize cats in images would be trained on multiple pictures of cats. During training, the model would identify consistent features in those images that it then would attempt to detect in new test images to determine whether they contained a cat. The model acts as a black box, meaning it does not explicitly spell out the criteria it has learned; one caveat is that this can hide biases and lead to erroneous results.
In a recent paper published in Nature Communications, a team of researchers from IBM Research — which boasts a robust AI research profile and hosts a free, cloud-based AI tool for digital chemistry projects — introduce a machine-learning tool that could help chemists identify suitable enzymes for industrial applications. Their model incorporates knowledge of enzyme–substrate interactions from a vast training set called ECREACT created by pooling together four preexisting databases. To boost their model’s chemical acumen, the authors also added one million organic reactions contained in the U.S. Patent Office database to the training regimen. Although these reactions differed from the enzyme-catalyzed ones, their addition helped the model better grasp how molecules interact in both enzyme-catalyzed and noncatalyzed reactions. They also included both forward and backward prediction capabilities in their model to enable the prediction of products from a given enzyme and substrates (forward) and of substrates and corresponding enzymes from a given product (backward).
Their final model could perform forward and backward prediction with good accuracy, suggesting that it successfully had learned the specific features of the substrates and products of each enzyme class. However, as with any machine-learning model, the results were biased by the quality and quantity of the training data; enzyme classes with sparse input data suffered from subpar prediction accuracy in either direction. The authors also noted that their training data was comprised primarily of biosynthetic reactions with natural products and substrates, resulting in a bias that could hinder those searching for synthetic routes for nonnatural products or seeking to use nonnatural substrates. Despite these limitations, the model — openly available for others to use as-is or with additional training (for example, on proprietary data sets) — represents an important step forward in the quest to use enzymes for greener chemistry.
Enjoy reading ASBMB Today?
Become a member to receive the print edition four times a year and the digital edition weekly.
Learn moreGet the latest from ASBMB Today
Enter your email address, and we’ll send you a weekly email with recent articles, interviews and more.
Latest in Science
Science highlights or most popular articles
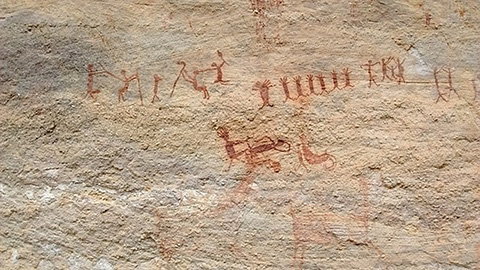
Liver enzyme holds key to adjusting to high-protein diets
Researchers at the University of Geneva show that glutamate dehydrogenase controls blood alkalinity during fasting.
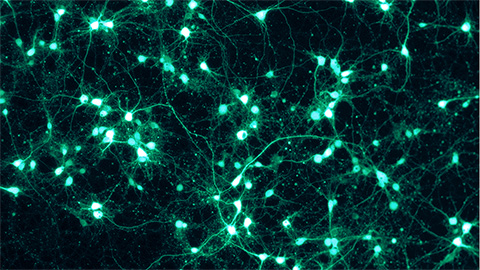
Adults grow new brain cells
How does the rare birth of these new neurons contribute to cognitive function?
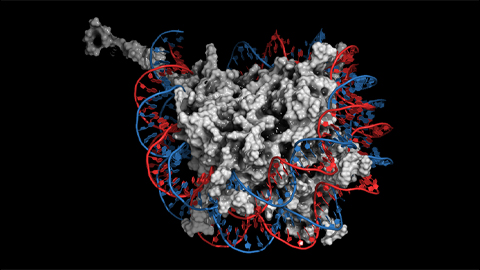
From the journals: JBC
Histone demethylase inhibited by own sequence. MicroRNA reduces cell cycle–related apoptosis. Multipurpose antibiotic takes on staph infections. Read about recent JBC papers on these topics.
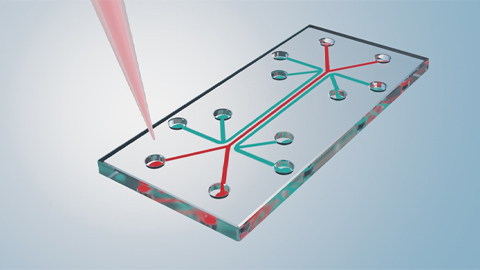
Tiny laboratories that fit in your hand can rapidly identify pathogens using electricity
Pathogens have distinct electrical charges, shapes and sizes. Measuring how quickly they move through an electric field can help researchers separate different species in a sample.

Toxoplasma gondii parasite uses unconventional method to make proteins for evasion of drug treatment
This recent study by a team from Bill Sullivan’s lab at the Indiana University School of Medicine was named a Journal of Biological Chemistry Editor’s Pick.
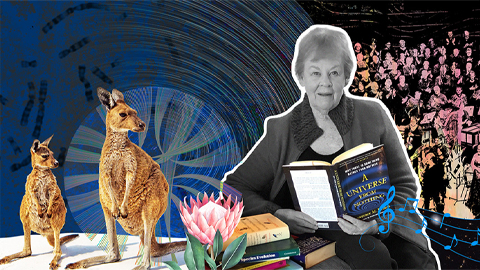
Of genes, chromosomes and oratorios
Jenny Graves has spent her life mapping genes and comparing genomes. Now she’s created a musical opus about evolution of life on this planet — bringing the same drive and experimentalism she brought to the study of marsupial chromosomes.