I took the NIH implicit bias training
As a Black queer woman with a biomedical Ph.D. who advocates professionally for the concerns of other scientists, in particular those in groups that have been historically marginalized in STEM, I decided it made good sense for me to complete the training too.
Though I had never had such training before, I’d experienced discrimination and I wanted to know what the NIH thought the scientific community should know about bias and how that aligned or differed from what I thought the community should know about bias.
While the training certainly raises awareness of various types of biases, in my view, it falls short in tackling explicit biases in STEM.
About implicit bias
Implicit biases are the prejudices and stereotypes that influence our decision making without us realizing it. These biases cause us to judge, exclude, and cause harm to individuals that we have stereotypes and prejudices against leading to inequity in our personal and professional lives.Over the years, scholars have debated on the effectiveness of mandatory unconscious bias training and if it has any impact on overturning individual biases. A general consensus is that only requiring employees to take training is not enough. Real change comes from training along with tools and strategies to assist in individual and organizational behavioral change.
About the training
The NIH’s two-hour interactive training offers evidence-based information regarding different types of biases, such as affinity bias, confirmation bias and group think.It includes fictional scenarios depicting different biases highlighted in the training, and tests your ability to reflect on your own biases through multiple choice self-assessments.
Overall, I found the training to be informative and thought provoking, as it challenged my own implicit biases. For example, it made me notice that I find biases in others while failing to see historically marginalized groups that I’m unfamiliar with, such as religious minority groups, as being underrepresented in STEM.
However, if NIH wants to counter biases specific to the biomedical science enterprise, it must contend with the power dynamics that exist at the agency and in academia. The modules failed to provide examples of these power imbalances, especially pertaining to historically underserved trainees.
Nor did it provide examples of experiences of investigators with diverse gender identities, religious beliefs or disabilities. It did not touch on ageism or delve into known forms of discrimination that occur at NIH and its funded institutions, such as sexual harassment, lack of diversity in high-level positions and grant funding inequalities.
Furthermore, as important as it is to raise awareness of implicit biases, the truth is that NIH needs to deal with explicit biases.
In 2020, the NIH launched the UNITE program to end structural racism at the agency and the biomedical research enterprise.
UNITE has five committees with the following specific aims:
- U — Understanding stakeholder experiences through listening and learning
- N — New research on health disparities, minority health, and health equity
- I — Improving the NIH culture and structure for equity, inclusion, and excellence
- T — Transparency, communication, and accountability with our internal and external stakeholders
- E — Extramural research ecosystem: changing policy, culture, and structure to promote workforce diversity
While addressing implicit biases is a step toward countering racism and discrimination against historically marginalized groups, there need to be efforts in combating the explicit biases that drive intentional acts of discrimination.
In order to end structural racism and discrimination, the core problems of racism, ableism, and misogyny must be addressed. Explicit biases are both the creation and result of racist and prejudicial policies enacted to uphold racial hierarchy and unbalanced power dynamics. Until explicit biases are dismantled, structural racism and discrimination will continue to persist.
Overall, I found that although the pros of the implicit bias training outweigh the cons in terms of number, there is much room for improvement.
Here are the pros. The course:
- Gives examples of how implicit bias affects NIH.
- Challenges participants’ viewpoints on personal biases.
- Has closed captions and is accessible.
- Incorporates self-assessment for areas of growth.
- Displays examples of biases in hiring practices.
- Lacks examples of implicit bias in mentoring, and offers only one example of bias in peer review.
- Does not explore implicit biases relating to gender identity (beyond the gender binary), sexual orientation, age or disability.
Click HERE to learn more about the NIH Implicit Bias training.
Enjoy reading ASBMB Today?
Become a member to receive the print edition monthly and the digital edition weekly.
Learn moreGet the latest from ASBMB Today
Enter your email address, and we’ll send you a weekly email with recent articles, interviews and more.
Latest in Policy
Policy highlights or most popular articles
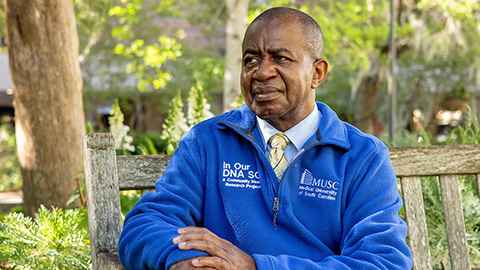
Genetics studies have a diversity problem that researchers struggle to fix
Researchers in South Carolina are trying to build a DNA database to better understand how genetics affects health risks. But they’re struggling to recruit enough Black participants.
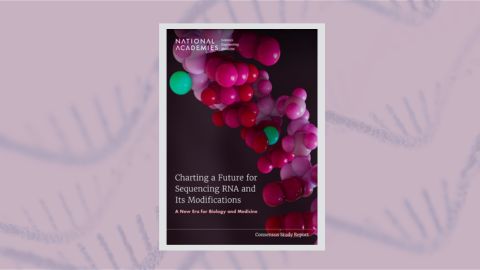
National Academies propose initiative to sequence all RNA molecules
Unlocking the epitranscriptome could transform health, medicine, agriculture, energy and national security.
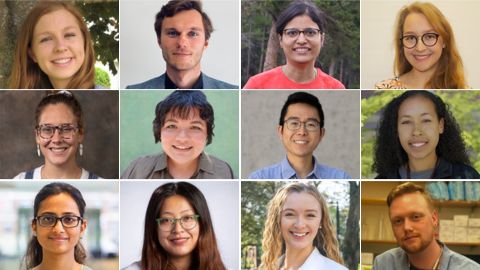
ATP delegates push for improved policies
This ASBMB program helps advocates gain skills to address issues that affect science and scientists.
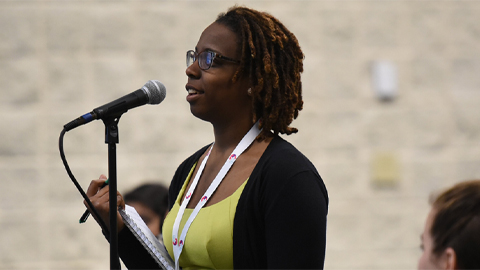
Advocacy workshops at Discover BMB 2024
Topics include running for office, becoming an advocate, and navigating the grant review process at the NIH.
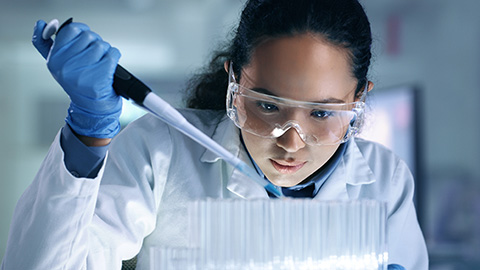
NIH’s advisory committee releases report on re-envisioning postdoc training
The working group developed six primary recommendations for the National Institutes of Health.
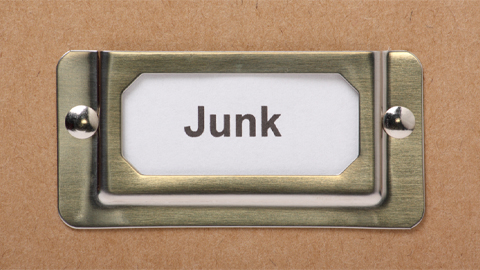
When authoritative sources hold onto bad data
A legal scholar explains the need for government databases to retract information.